I met one of my heroes.
Perhaps ‘hero’ isn’t the perfect word to best describe my view of Stuart A. Kauffman. But it comes close. Suffice it to say that I’ve been a fanboy of Kauffman for well over a decade, and the chance of meeting him was one of the top three reasons for my attending the Science of Consciousness Conference (TSC) recently held in Tucson, AZ.
Maybe you are not familiar with the Santa Fe Institute (SFI), where Kauffman was faculty in residence. The SFI, founded in 1984, has been instrumental in goading the cross-disciplinary study of complex adaptive systems. Kauffman led those efforts during the first important decade of that work. Maybe you don’t know what a MacArther Fellowship is — it’s the ‘Genius Grant’ — a substantial prize awarded annually to a few dozen exceptional individuals in a variety of fields. Kauffman was a MacArthur Fellow from ’87 through ’92. But surely you’ve heard of Darwin. You know what economics is. You probably have some appreciation for computer design, genetics, and even quantum mechanics — at least on a science fiction level.
Well, then you should know that as a theoretical biologist, medical doctor, complexity studies pioneer, and profound influencer of thought, Stuart A. Kauffman is one of the giants of our age, having influenced how we now think about evolution, economics, and even computer programming. And I got to meet him.
I first came across his work in the early part of this century. Liz and I were living in Manhattan at the time, and my work at Sun Microsystems required copious trips on international flights, all of which gave me ample time to read. Kauffman’s book Investigations was one of many that drew my attention.
But I was so taken with his ideas that I had to read it twice. And then, giving Kauffman full credit for his ideas, I refactored many of his concepts in my own investigations in distributed computing. Ideas like autonomous agents, emergence, and the adjacent possible all found their way into my own text on distributed computing which was published a few years afterward. In fact, looking back, I believe reading Kauffman helped me formulate many of my own ideas and gave me impetus and the drive to dare to write an ambitious book of my own.
Here we are 15 years later, and I’m a bit of an international traveler again, with flights and miles of time to read. Instead of evangelizing Java, I’m teaching Data Science and Big Data technologies for Think Big Analytics, a Teradata Company. But I am again prone to read more with opportunities to do so. My old friend of mind came to mind, so I shopped for any new works by Kauffman. And there it was — something very new. In fact, it was about to be published as I prepared for a recent 6-week trip to Prague and Mumbai earlier this year, Kauffman’s latest: Humanity in a Creative Universe. So I grabbed a Kindle copy and consumed with glee this new work from my old friend.
Regarding his new book, first, you absolutely must buy it. Then read it. Read it again. Kauffman suggests ideas and experiments that must give us pause. Quantum physics allows brief glimpses through a glass darkly into a baffling, non-intuitive universe. We sort of understand the mathematics of than quantum view, but cannot agree on meaning. There are dozens of interpretations of quantum mechanics. We are still just beginning. The foundation of everything we think we know was fundamentally rocked by much of 20th century science. Quantum physics is perhaps the most essential part of that, but its best gifts are yet to come.
All of the drivers of modern technology, and therefore civilization, were enabled by 20th century discoveries. Every endeavor of human life now reflects that work — communications, energy, education, entertainment, finance, manufacturing, medicine, transportation, technology…even religion. Our world (universe) view has metaphorically and quite literally exploded, expanding at an accelerating rate as is the cosmos itself. It was Maxwell, and Einstein and Hubble and Gödel that did it. Schrödinger and Shannon and von Neumann and Turing, and Watson and Crick, and a host of others. Giants upon whose shoulders we stand. That tectonic shift of mind was due to those 20th century giants and the gifts those giants left us. But even with all those changes, the sum of which ultimately gave rise the the Network Age, many 20th century discoveries are only now starting to yield engineered fruits. Quantum physics is that.
I cannot do Kauffman’s latest tome justice here. But there is something he does posit with fresh eyes that deserves a viewing in the theatre of human discovery: the Poised Realm.
The Poised Realm
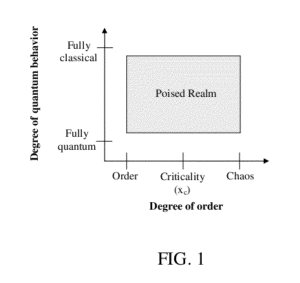
From a deeper exploration of the adjacent possible, Kauffman mixes a cocktail of theoretical biology, complexity studies, and quantum mechanics to suggest an ontologically real realm that is both quantum and classical, existing in-between. I will leave it to Kauffman to provide the evidence, for which there are compelling arguments. But if we stipulate that Kauffman’s Poised Realm exists, in a neo-Cartesian sense, cleverly fitting between Res Potentia (that which is possible) and Res Extensa (that which is actual), then perhaps consciousness is the bridge; the Mind and life itself, poised critically as quantum coherent/de-coherent/re-coherent engine, providing the qualia; the magic. Human consciousness.
Yeah. That’s a lot to think about: consciousness.
Consciousness
The thing is, each of us knows we have it, but sometimes we are not so sure about everybody else. Who among us hasn’t bellied up to the bar at the Solipsism Saloon at some point and lapped up a few? The truth is, Descartes put us all on that path, cogito ergo summing us all into a post-modernity built on the foundations of mathematics, science, and exponential technological growth — heavily infused with deep alienation and a throbbing ipseity hangover.
But that particular elephant in the room — the hard problem of human consciousness — is today something we can quietly discuss and share in our cloistered safe spaces. It is now something okay to perhaps even study, when once it was akin to sporting a voodoo facial tattoo at a Mormon picnic, risking marginalization and intellectual banishment.
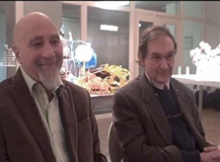
Stuart Hameroff and Sir Roger Penrose
For the past 20 years
Stuart Hameroff (of OrchOR fame) has been one of the leaders on the daring trek to a land which heretofore only artists and undergraduates would dare roam in the spooky underground of absinthe soaked nights. Hameroff is an anesthesiologist by profession, and another (albeit more recent) hero of mine. What better place to stand than anesthesiology when it comes to studying the on/off switch of awareness?
Meandering through this post, I am coming to the one salient question I attended the TSC conference to ask. In addition to meeting Kauffman and Hameroff — remember I said there were three top reasons for me heading for Tucson — I had a burning question. In his most recent work Kauffman discussed Trans-Turing Systems, the thought of which exploded in my head like the genetic memory of some Big Bang.
Trans-Turing Systems
From the Poised Realm, the embodiment of Trans-Turing Systems, as a real invention, doth flow. Of course you are familiar with the Turing Machine: the theoretical paper tape compute engine to which all modern processors are obliged to worship every Sunday…you are, of course, familiar with the Turing Machine.
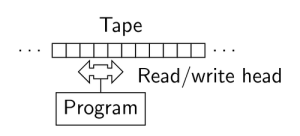
The Turing Machine
The work of Alan Turing is the rock from which the quest for a congruent theoretical computer science was launched. Totally awesome quantum computing heavy
Scott Aaronson has written that when it comes to AI, we can divide everything that’s been said about it into two categories: the 70% that was covered in Turing’s 1950 paper
Computing Machinery and Intelligence, and the remaining 30% that has followed in the decades since then.
Turing Machine = Foundation of Computer Science
So whoa — a Trans-Turing System? What?? I must know more!
That was the third thing that drove me to Tucson. I had to ask Kauffman what he meant — what he saw, what he imagined. I found a description in the patent that Kauffman, et al, filed in 2014:
"Further disclosed herein is a Trans-Turing machine that includes a plurality of nodes, each node comprising at least one quantum degree of freedom that is coupled to at least one quantum degree of freedom in another node and at least one classical degree of freedom that is coupled to at least one classical degree of freedom in another node, wherein the nodes are configured such that the quantum degrees of freedom decohere to classicity and thereby alter the classical degrees of freedom, which then alter the decoherence rate of remaining quantum degrees of freedom; at least one input signal generator configured to produce an input signal that recoheres classical degrees of freedom to quantum degrees of freedom; and a detector configured to receive quantum or classical output signals from the nodes."
Sweet. I got it. Quantum computing nodes working in tandem with classical compute (Turing Machine) systems and what emerges is a Trans-Turing Machine, not constrained nor otherwise entailed by a bothersome set of NP-complete limits. Polynomial hierarchy collapse ensues, at long last P = NP, and we are full throttle to ride warp drive engines to the stars! Maybe? Maybe. Maybe not.
I had to ask Kauffman.
After I spotted him at the outdoor mixer on Thursday night, after I got over my fanboy flutters, after I introduced myself, chatted with him for a bit about his new book and how much I liked it, after I explained my own thoughts from my field in computer science, and how his book from a decade and a half earlier had so deeply influenced me, I did finally ask.
“So how do we build the Trans-Turnig Machine?”
A wry smile crossed his face. His eyes lit. For a moment he stopped being the intellectual giant I had come to revere, and revealed the mischievous, inquisitive, childlike spirit that must have driven him his entire life.
“I have no idea,” he said replied with a grin.
I was all satisfied. I knew he did not mean that he could not conceive of one, nor he did mean that he could not describe one, nor not define the attributes it might require, nor not imagine how it might function. What he meant was we still don’t know enough about quantum computing to imbue an instrument of our own creation with something akin to consciousness — whatever that means.
Today we all harvest the ample fruits from the first baby steps into the Network Age. We are still painting a digital patina over the planet. More stuff soon will think. We are clearly well into the age of pervasive computing, but computing is not yet ubiquitous, though soon it will be. Soon — within a decade — everything will be engineered to connect with everything, and almost all those systems are and will be awesome Turing Machines, programmable systems all, that will link us all together in a transcendent fine-grained meshed digital fabric of increasing value. Yet on the fringes, there is quantum computing, playfully peeking through from behind the classical physics curtain. And therein lies the unpredictable. It could be that Here There Be Monsters. Or not. That’s the beauty and the bizarre of where we are. Both terror and elation are on the rise, though neither are as appropriate nor as compelling as is the raw, robust curiosity that drives us ever forward.
Is the ineffable thing to come a D-Wave progeny? Maybe. Will Scott Aaronson explain and extend the exploding adjacent possible? Probably. Did Kauffman and Hameroff lead us to the brink? Absolutely. And from the wily Trans-Turing Machine, will Machine Consciousness one day emerge … whatever that means?
I have no idea.