Quadratic Bowling
In November of last year I conducted a simple experiment, wondering if activity level on github could be a predictive factor in open source framework adoption. So I grabbed data from github APIs from several large organizations and played with the data in RStudio. Projects from AWS, Google, Apache, Facebook, LinkedIn, Microsoft, Oracle and Twitter were all included in the analysis, My intent was to find the most active projects and determine if those projects were increasing or decreasing in activity over the previous 12 months.
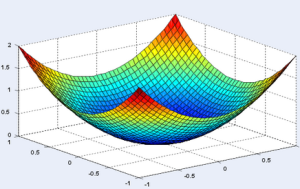
In the end, nothing terribly insightful emerged. If anything, the results underscored my own intuition as to where things would go in 2016. Hadoop, Cassandra, and Ambari all exhibited strong, steady interest. Spark was growing rapidly. As it turns out, Spark adoption in 2016 appears to be strongly correlated to github activity level increases in 2015, as Hadopp continues to find harbor in organizations of all sizes. But it didn’t take a data scientist to figure that out. Any reasonably-aware industry professional would have reached a similar conclusion.
I may or may not repeat a similar experiment this coming November. My instincts tell me that Machine Learning, including Neural Networks, will exhibit rapid adoption in 2017/2018, like that of Spark in 2016. Indeed, one of the motives for Spark adoption is to enable Machine Learning commercialization.
But just as adoption of Network Age technologies gave rise to major economic shifts at the turn of the century, the coming next phase (which includes pervasive adoption of IoT, good old fashioned AI (GOFAI), VR/AR, and a slew of sensors) will be an economic tsunami.
Evidence of GOFAI adoption is pretty much weekly news at this point in history. One notable story in particular from the headlines of this past week is the Google AI that invented its own crypto for messaging. Sure, it smacks of Terminator/Matrix darkness. But in reality, such innovation might very well be the foundation upon which the cyber-security of 2020 will rely.
As you know, the rapid rate of change we witness today is based on logarithmic accelerations of capabilities in Information Technology of which Moore’s Law is an exemplar. With each doubling of CPU cycles, storage capability and data itself, we brave frontiers increasingly riddled with attractors of both hope and terror. As prudent technologists, we must expect more Network Age asteroids. Dinosaurs beware. Mammals prepare. That’s what my instincts tell me now.
With the commercialization of GOFAI the quadratic bowl, as metaphor for Machine Learning artifact, might be just the thing for geek holiday gifts. Representing the error surface of the linear neuron in Neural Network implementations, for a linear neuron with a squared error, the visual (and mathematical) abstraction is a quadratic bowl. Too bad the Etsy shop in question seems to be taking a break.
Beyond Machine Learning uptake in 2017, other predictions based on GOFAI commercial adoption naturally follow. For example, the next 10,000 startup business plans will add Machine Learning to something extant — some process, some flow, some problem set. Lower costs, increase speed, provide more for less by applying AI. That sort of incremental approach to innovation is probably the most common and likely. There is another set too — the disruptor flavored startups. Those innovations we cannot predict given the infinite adjacent possible. But we can prepare for asteroids, if mammals we would be. I’ll write more on that later.
Happy quadratic bowling.